New research shows how human-aware AI helps us accelerate scientific discoveries
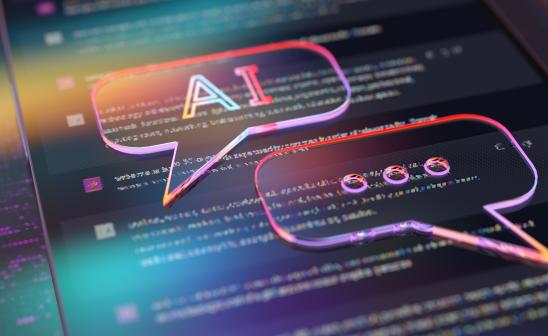
"It's about changing the framing of AI from artificial intelligence to radically augmented intelligence.”
A new study explores how artificial intelligence can not only better predict new scientific discoveries, but also to usefully expand them. The researchers, who published their work in Nature Human Behaviour, built models that could predict human inferences and the scientists who will make them. The authors also built models that avoided human inference to generate scientifically promising "alien" hypotheses that would not likely be considered until the distant future, if at all. They argue that the two demonstrations — the first allowing for the acceleration of human discovery, while the second identifies and passes over its blind spots — means that a human-aware AI would allow for movement beyond the contemporary scientific frontier.
“If you build in awareness to what people are doing, you can improve prediction and leapfrog them to accelerate science,” says co-author James A. Evans, the Max Palevsky Professor in the Department of Sociology and director of the Knowledge Lab. “But you can also figure out what people can't currently do, or won't be able to do for decades or more into the future. You can augment them by providing them that kind of complementary intelligence.”
AI models that have been trained on published scientific findings have been used to invent valuable materials and targeted therapies, but they typically ignore the distribution of human scientists involved. The researchers considered how humans have competed and collaborated on research throughout history, so they wondered what could be learned if AI programs were explicitly made aware of the human expertise: Could we do a better job of complementing the collective human capacity by pursuing and exploring places humans haven’t explored?
To test the question, the team first simulated reasoning processes by building random walks across research literature. They began with a property, such as COVID vaccination, then jumped to a paper with that same property, to another paper by the same author, or a material cited in that paper. They ran millions of these random walks and their model offered a 400% improvement of predictions of future discoveries beyond those focused on research content alone, especially when relevant literature was sparse. They could also predict with greater than 40% precision the actual people who would make each of those discoveries, because the program knew that the predicted individual was one of only a few whose experience or relationships linked the property and material in question.
Evans refers to the model as a “digital double” of the scientific system, which allows simulation of what is likely to happen in it, and experimentation of alternative possibilities. He explains how this highlights the ways in which scientists hew close to the methods, properties, and people with which they have experience.
“It allows us to also learn things about that system and its limits,” he says. “For example, on average, it suggests that some aspects of our current scientific system, like graduate education, are not tuned for discovery. They're tuned for giving people a label that helps them get a job — for filling the labor market. They do not optimize discovery of new, technologically relevant things. To do that, each student would be an experiment — crossing novel gaps in the landscape of expertise.”
In the paper’s second demonstration, they asked the AI model not to make the predictions most likely to be discovered by people, but to find predictions that are scientifically plausible, but least likely to be discovered by people. The researchers treated these as so-called alien or complementary inferences, which had three features: They’re rarely discovered by humans; if discovered, it won’t be for many years into the future when scientific systems reorganize themselves; and the alien inferences are, on average,better than human inferences, likely because humans will focus on squeezing every ounce of discovery from an existing theory or approach before exploring a new one. Because these models avoid connections and configurations of human scientific activity, they explore entirely new territory.
Evans explains that looking at AI as an attempt to copy human capacity — building on Alan Turing’s idea of the imitation game where humans are the standards of intelligence — does not help scientists accelerate their ability to solve problems. We're much more likely to benefit from a radical augmentation of our collective intelligence, he says, rather than an artificial replication.
“People in these domains — science, technology, culture — they're trying to stay close to the pack,” Evans says. “You survive by having influence when others use your ideas or technology. And you maximize this by staying close to the pack. Our models complement that bias by creating algorithms that follow signals of scientific plausibility, but exclusively avoid the pack.”
Using AI to move outside existing methods and collaborations, rather than reflecting what human scientists are likely to think in the near future, expands human capacity and supports improved exploration.
“It's about changing the framing of AI from artificial intelligence to radically augmented intelligence, which requires studying more, not less, about individual and collective cognitive capacity,” Evans says. “When we understand more about human understanding, we can explicitly design systems that compensate for its limitations and lead to us to collectively know more.”